Different cultures see the world through unique lenses, especially when it comes to colours. Take the Eskimo, for example, who distinguish between 100 types of snow, while others might simply see it as “white stuff.” This diversity in perception has intrigued scientists for years, spanning across fields from cognitive science to anthropology.
Now, researchers at RIKEN AIP are tackling this puzzle from a fresh angle: machine learning. For the first time, they’re asking whether artificial intelligence (AI) can develop colour perception similar to humans. This is research is selected as oral presentation with an oral presentation (1.8%) at the top AI conference International Conference on Computer Vision (ICCV 2023). This will lay the foundation to develop next generation of large language model (LLM) by creating tokenization phase from an evolutionary approach.

Fig.1 World Color Survey stimulus palette for Nafaanra in 1978 and 2018.
Imagine this: Human languages evolve over time to include new colour names, driven by the need for efficient communication. As shown in Fig.1, a study called the World Colour Survey collected data from speakers of 110 different languages around the globe. Over time, languages like Nafaanra in northwest Ghana have expanded their colour vocabulary from three in 1978 to ten in 2018 to keep up with the modern world, as seen in Fig.1.
But can machines undergo a similar evolution? The researchers at RIKEN AIP set out to find out. As shown in Fig .2 , they developed a method which simulates the efficiency of communication by simplifying the colour space, which used to be 2563 ,into just a handful of colours, while still maintaining accuracy. Surprisingly, this method achieved remarkable results, with a high-level recognition accuracy of 50.6% on the CIFAR100 dataset using just two colours.
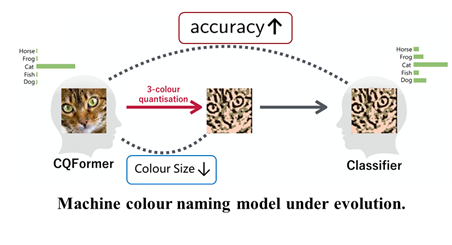
Fig.2. Illustration of machine colour naming evolving.
Even more fascinating, the trade-offs between complexity and accuracy observed in the AI system closely mirrored findings in linguistic research. As shown in Fig.3, the AI’s evolution of colour categories (b) followed similar patterns to those observed in human languages (a).
Moreover, when the system was introduced to the three-colour system of Nafaanra from 1978, it automatically evolved to include a fourth colour close to yellow-green, aligning with universal patterns observed in language evolution. Berlin and Kay found universal restrictions on colour naming across cultures and claimed languages acquire new basic colour categories in a strict chronological sequence. For example, if a culture has three colours (light (‘fiNge’), dark (‘wOO’), and warm or red-like (‘nyiE’) in Nafaanra), the fourth colour it evolves should be yellow or green, exactly the one (Fig. 3 (e)) discovered by the proposed system.
This breakthrough opens up exciting possibilities, especially for large language models (LLMs) that currently rely on specific languages like English or Japanese. By adopting an evolutionary approach, AI could develop optimised language systems independent of human languages, breaking new ground in the field of artificial intelligence.
In essence, this research isn’t just about colours; it’s about understanding how AI can learn and evolve in ways that mirror human cognition, leading to smarter, more adaptable technologies in the future.
- Paper information
Title: Name Your Colour For the Task: Artificially Discover Colour Naming via Colour Quantisation Transformer
Journal name: Proceedings of the IEEE/CVF International Conference on Computer Vision
Authors: Shenghan Su, Lin Gu (* corresponding author) , Yue Yang, Zenghui Zhang, Tatsuya Harada